H.R. Alerting system
In any organisation excessive staff turnover is a disruptive and expensive drag on proper functioning. In some industries with high training requirements and recruitment costs, it can be a major budgetary headache. Reducing staff turnover by intervening to solve problems before they result in staff leaving can lead to considerable savings.
The SemantEx system is currently being deployed with a social care provider to help solve this problem. A variety of documents are produced which can be analysed by the system, incident reports, end-of-shift logs and staff-manager interviews for instance. The system is setup to receive and graph these documents as they are produced and to alert management if any potential issues are detected.
So is this just sentiment tracking? Yes - but it is important to understand the advantages that the SemantEx system brings to this application.
The first and most important is precision. Because we are taking the language apart and extracting relationships, rather than just counting sentiment words and perhaps looking at term proximity, we can be understand what might be causing a change in sentiment not just that it is happening. The plot below illustrates this (real data with changed names):
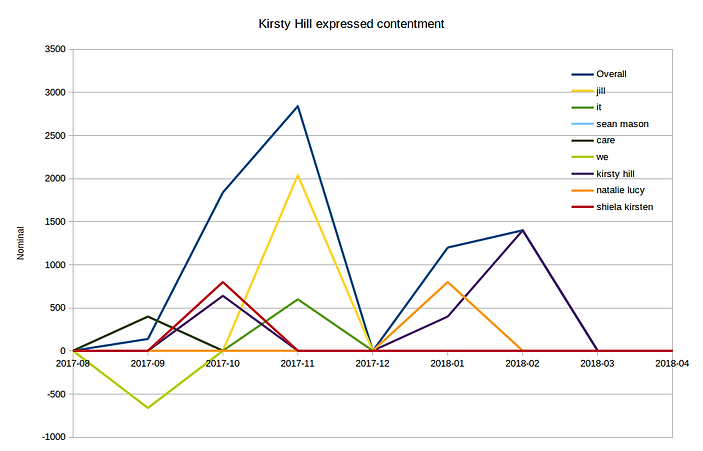
The second major advantage of the system is that it does not require vast amounts of historical data for training. It can start work from day 1. It is true that differences in the way people use language (some are more gushy and enthusiastic than others for instance) mean that differential expression (changes over time) are more useful than single point assessments. However it is also true that the system can detect the use of more hyperbolic language and include this in assessments if required.
The SemantEx system also has major advantages stemming from its deployment method. Typically it will live within an organisation's firewall meaning sensitive data need not be shipped to external non-owned servers. Secondly all the data transformations that lead to an alert and possible intervention are clearly understandable and easily traceable. This is a quite different scenario to a neural-net based system where, for instance, historically biased data disappears into the 'black box' and its affect on any outcome is unknowable and untraceable.
The project is still in the early stages but already has demonstrated that it can predict from training logs which staff are likely to be in post after 6 months - not with 100% accuracy but certainly usefully, enabling management to make assessments, about whether further training or role adjustment might improve outcomes.
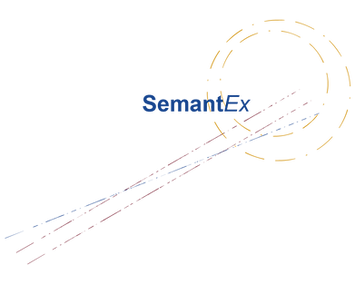